Are you smart enough (OR) ready to become SMARTER than ever before? If so, impose and implement Machine Learning in YOU! Don’t look at me, this is the most recent trend, greatest beneficial and most of them are adopting across all industries, so prepare for a transformation in your every action and process, this is discussion between Logistics, Warehouse, Transportation and Supply Chain Management.
This article will take us through this cutting-edge digital transformation technology and to see how we can apply the same inLogistics and Transportation Management(LTM) to increase business opportunities, efficiency and challenging your competitors in the runway of your business tracks.
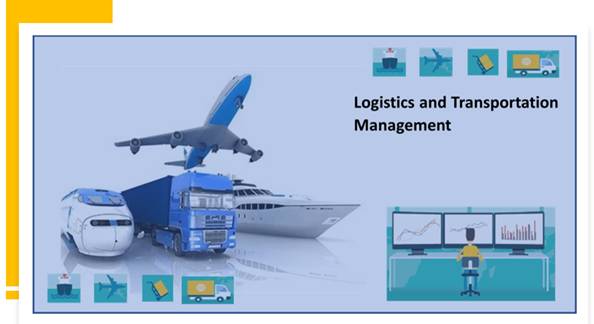
Let’s go through the fundamental definitions,
Logistics and Transportationare two highly significant process models that follow each other in goods transportation and distribution services.
The administration of the inward and outward conveyance of commodities from the manufacturer to the end user is referred to as Logistics.
Transportationis simply the movement of goods between two points or across a set of dropping points, each of which is labelled with the individual goods.
Most of the time, these two concepts are mutually consistent in the supply chain sector, and we can simply say that getting items and services from one point to another, after the product/goods have been sorted, packed, and labelled for dispatch.
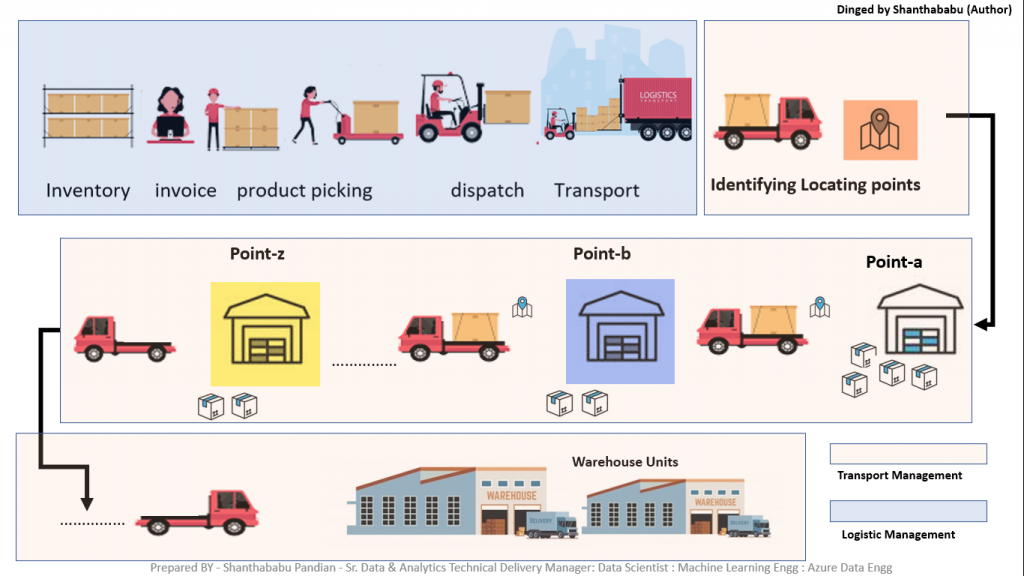
As seen in the preceding sequence, Logistics is responsible for procuring, packing, making invoices, loading onto the truck, and distributing supplies, goods, and products to an end customer.
While Transportation is responsible for transferring materials, goods, and products to an end customer. Both process models include a series of activities designed to improve the efficiency and effectiveness of materials/goods and services storage and transportation.
Let’s talk about Artificial Intelligence and how we could improve both services (Logistics and Transportation)with the help of this buzzword.
Artificial intelligence(AI): is the replication of human intellect practices using machines, specifically computers, to write a code and automate the same in a very clever way of thinking for itself and making decisions.
Why is AIrequired?
AI makes a difference in completing tasks as we used to do manually and without errors (99%). A pre-planned list of tasks is handled in a quicker, accurate manner and depending on the circumstance and the procedure. It undoubtedly improves the existing process model and way of doing things in any kind of industries and its business development by solving difficult problem statements that were extremely difficult to execute manually.
Since the AI system has been developed and trained in such a way that it can handle most of the scenarios. In the next section of this article, we will go over how AI may be used to improve Logistics and Transportation Management (LTM).
Where can AI be found? Yes, ANYWHERE.
Automation differs from AI, Automationis based on business rules and a series of actions that have already been specified in the system,simply we could say set rules in MS-Outlook. However, AI has more intelligence and designed to learn and make decisions based on its own experience and continuously improving process model and more stable, certainly AI is Dynamic. AI is transforming all any of industries, the below picture is the evidence for the same.
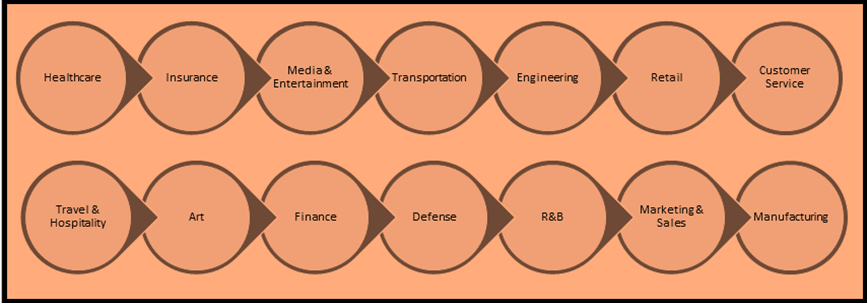
AIML (Artificial Intelligence and Machine Learning) has been more important in every business in recent years, and the Logistics and Transportation arena is no different. Before we get into AIML in Logistics and Transportation, let us talk about the processes on both sides and connect them with AIML.
What is the optimal Logistics System and process model?
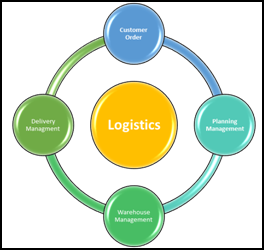
The logistics industry expects and/or requires the following AI applications to improve business.
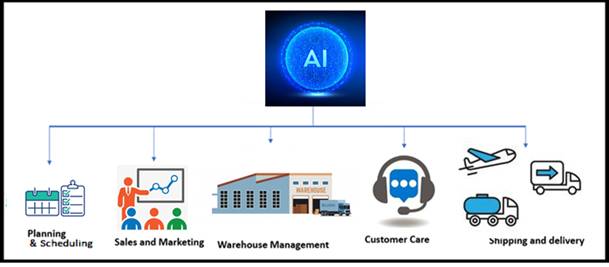
As we all know, sub-divisions are critical in logistics, as illustrated in the above image, and certainly AI scope in divisions.
Planning & Scheduling
Planning and Scheduling are the most important and fundamental requirements for any type of industry to meet market demand and have the capacity to do so in the future. This should be harmonized in order to maintain an effective set of processes and put in place an effective supply chain platform, where goods might be anything.
For their difficulties and new solutions, Supply Chain Planning must trust and utilize AIML models. According to Gartner, by 2023, AIML methodologies and its algorithm will be included in 25% of all supply chain-based technology-based solutions.
AIML clarifies different patterns in data collection from various data sources in supply chain platforms, using data wrangling, data cleaning, and applicable feature engineering approaches, as well as perfect model selection based on the nature of collected data and implementation of those, and improves logistics processes at various levels.
AIML offers the ability to allow logistics companies to use real-time data in their demand and forecasting models. Machine Learning models like Timeseries-based models are ideal for the supply chain domain.“Moving Average, ARIMA, and other time-series models“ are among them.
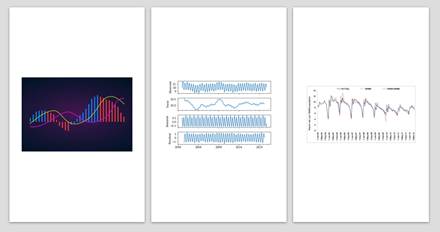
ARIMA (Auto-Regressive Integrated Moving Average)
Warehouse Management is part of logistics management.Nature of process and operation’s models aredependenceon the goods/products, what we are handling in the Wearhouse. Existing WMS (Warehouse Management System) is a set application that helps warehouse managers to monitor and handle the day-to-day tasks of a warehouse. All these WMS helps each manufacturing industries to control and observe 3rd party logistics, those who are helping the primary Warehouse. Certainly, we must have the integrated analysis of WMS, and extract the analyse as an output by applying ML models. All these models are very important for evaluating the performance of the Warehouse as it regulates the success or failure of the industry. For sure these models would help Warehouse management team to enhance the system and leading into bringing SMART Warehouse.
Let’s have a quick overview of “SMART Warehouse”.
Adoption of Internet of Things (IoT) in Warehouse is perfect solution of validating the prospects of the technology in various real-time scenario. The IoT technology helpswarehousemanagers to fulfil the expanding demands in order to sustain the company and scalingup successfully. And below is the list of benefits for IoT adoption. Ultimately the data are generated by IoT devices that are stored and processed for ML implementation.
- Predictive Maintenance of Warehouse
- Drafting Supply Chain Visibility
- Preventing Measures of Asset management and goods spoilage
- Figuring out cost effective and quicker delivery strategy
- Identifying Innovating automating tasks and improve the employee productivity.
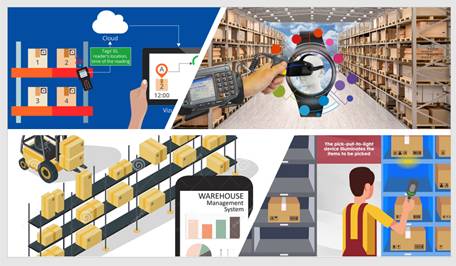
SMART Warehouse (IoT Enabled)
Transportation Management (TM)
As previously noted, numerous modes of transportation are available for the movement of goods from one area to another, and this TM is paired with Logistics services. In which goods/products can be transported by road, rail, sea, air, pipeline, and so on.
The performance and efficiency of transportation management can be analyzed for the best shortest delivery route, connecting various dropping locations for quick and cost-effective dispatch, as well as ensuring the safety of goods and instilling customer’s confidence in bulk or unit-based product delivery.
In today’s world, the TM has been improved at several levels by using technology and processes, such as creating and determining the shipment process methodology based on geographical location and the nature of the goods/products delivered.
The most significant innovation in this sector is the ability to anticipate Estimated Time of Arrival (ETA), track the vehicle, and use this information to manage multimodal transportation. Yes! Of course, with the assistance of Machine Learning algorithms and exceptional AI characteristics.
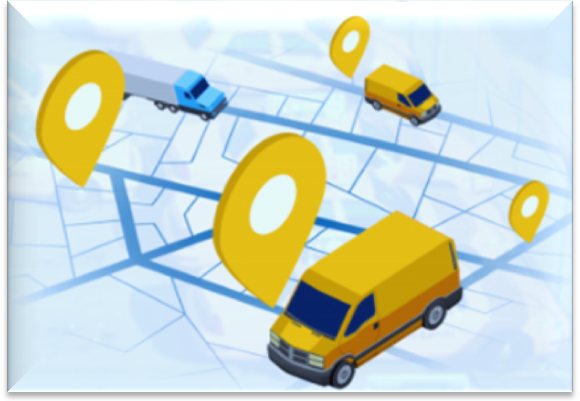
ETA prediction
PreciseETAprediction is of great value in transport management,to drive this model we need to have data from the past and present on-going delivery, and data which can be generated with help of various sensors connected with the vehicles (Smart vehicles/Connected vehicles) andbased on the collected data the model would work toward prediction methods.
Various Machine Learning (ML) algorithms are well suited to solve problem statements, sincelots of data variations in the collected data point, we could apply Linear and Non-Linear regression, Support Vector Regression (SVR/SVM) for that analyze data specifically for classification and regression analysis.
We were able to achieve deliveries in advance and material stock forecasting with the aid of AIML methodologies. Furthermore, a Warehouse raw materials capacity inventory in time, workers shift planning, equipment downtime planning, and raise its efficiency correspondingly.
Let’s go through the ML procedure a little more than halfway through this article.
Machine LearningProcess Model (Applicable for LTM)
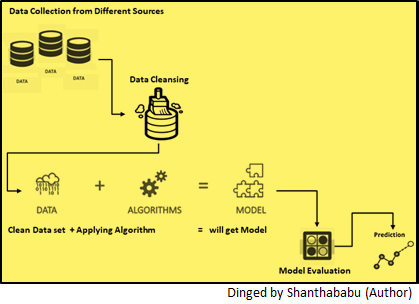
Sources for Data Logistics and Transportation Management (LTM):Data Source is key factor for any analysis,analytics, and machine learning culture.It would be history data, recent data and some cases streaming data. As well source for ML process, with respect to Logistics andTransportation below are the data source at minimum level. Each source has its own solutions for various problem statement across in LTM.
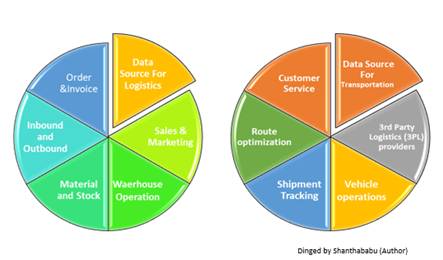
Sources for Data Logistics and Transportation Management
Data Cleaning and Waggling Process
Data wrangling process follows a set of steps which starts with extracting raw the data from the data source which we have mentioned above. In this process “munging” the raw data, parsing the data into required data structures, and finalizing the dataset and getting into Feature Engineering.
Model selection: is the primary process of picking one of the best models for a training dataset from a variety of models based on the nature of the dataset, the given problem statement, and the purpose of the suggested solution. Linear Regression, Logistic Regression, SVM, KNN, Random Forest, Decision Tree Model, and so on.
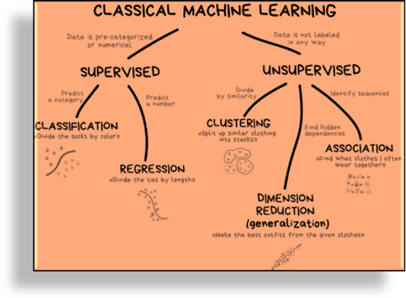
Machine Learning Classification
Simple Logistics and Transportation Management ML – Architecture
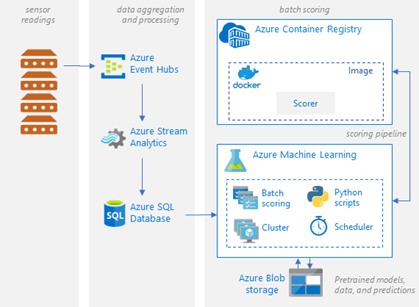
Author – Shanthababu.